Body
Current systems for controlling powered prosthetic legs require the user to manually transition the device between different walking modes. To go from level-ground walking to ascending stairs, for example, users must either perform specific unrelated body movements or use a key fob or a cellphone app to signal to the device that they want to change modes. These procedures make using the device unintuitive and laborious. When seated, the user must manually reposition their device for comfort or, for example, to transition into or out of a car.
Robotic powered prostheses have a number of built-in mechanical sensors that can gather information about the position, angle, and force exerted by the prosthetic joints. The information from these sensors can be analyzed by a computer algorithm that is able to learn and recognize patterns of sensor information generated during gait. Such pattern recognition technology can been used to accurately control powered devices.
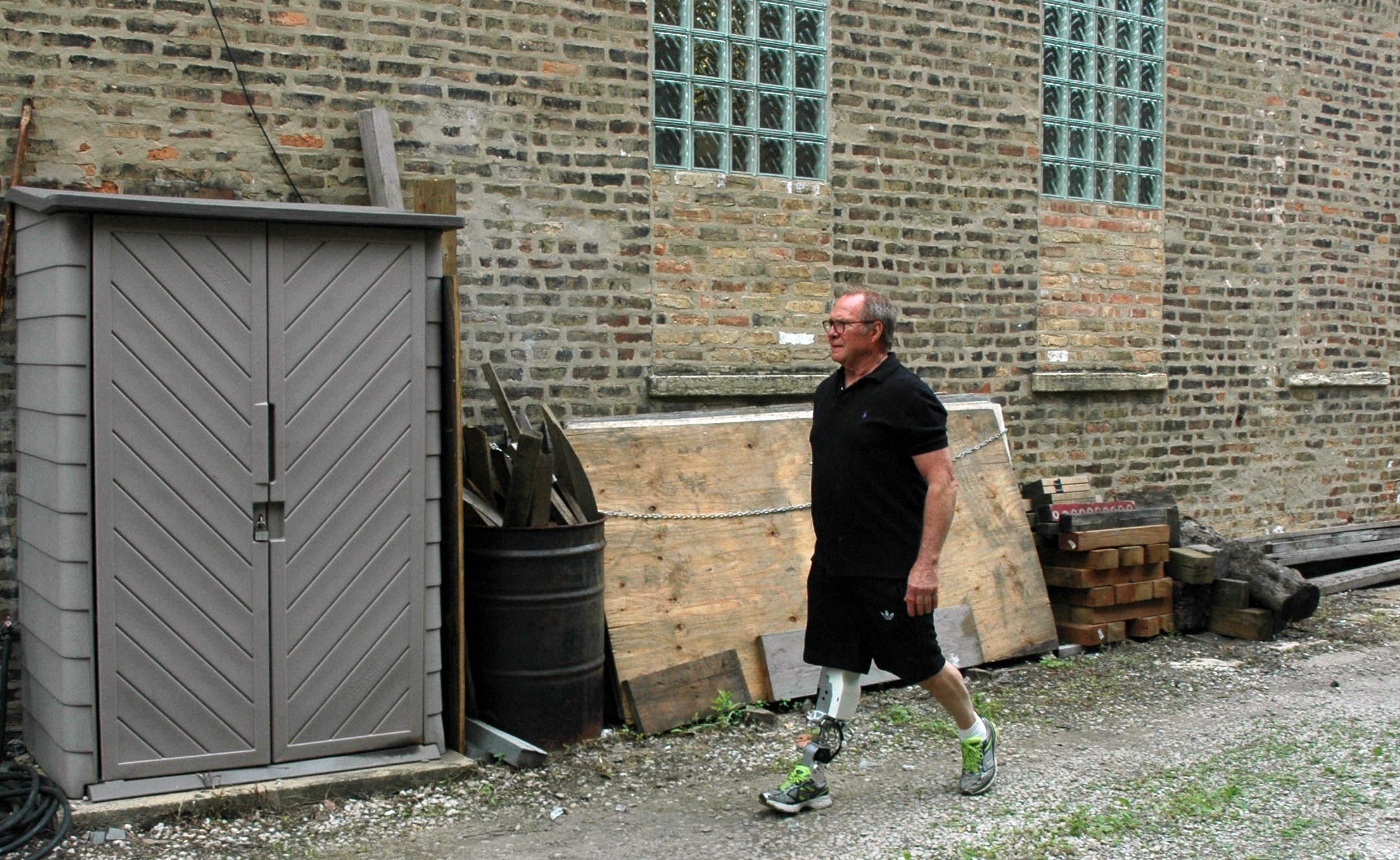
Moreover, these algorithms can also use neural information from the user to improve control of the device. When users move their residual limb to walk, their remaining muscles generate electrical signals (called electromyographic, or EMG signals). Similar to the mechanical sensor information, EMG data recorded from several muscles generates patterns that are specific to the type of movement being attempted. By combining EMG and mechanical sensor data, pattern recognition algorithms can recognize the patterns and control the prosthesis with far fewer errors. In addition, because EMG signals are generated a split second before movement is initiated, these signals can be used to predict upcoming transitions, enabling the user to seamlessly change to a different walking mode simply by attempting to do so—just like walking with an intact leg. Finally, when the user is seated, EMG signals alone can be used to reposition the leg as desired.
Our current research goals include refining the control system to reduce errors even further, developing algorithms that require less extensive training, and creating an adaptable algorithm that can not only learn to recognize signal patterns but can learn from previous classification mistakes, so that the system becomes more reliable over time.
Active Projects
Body
NIH R01HD079428-01
Adaptive Recalibration of Prosthetic Leg Neural Control System
Our goal is to understand what type of information is required to adequately adapt neural control systems for advanced powered prosthetic limbs. We will then use this knowledge to optimize an embedded system hardware capable of supporting adaptive algorithms being developed with a synergistic project (US Army W81XWH-14-C-0105).
NSF NRI
NRI: Small: Computational Motor Control for Better Control of Prosthetic Devices
The goal of this project is to a better understanding of the performance and limitations of existing prosthetic control systems within the principled framework of computational motor-control, and (ii) improved prosthesis control through the development of novel interface dynamics and haptic sensory cues.
US Army, W81XWH-14-C-0105
Configuration and Adaptive Recalibration of Lower Extremity Neural Control Systems
Our goal is to advance this research prototype, which requires daily recalibration, to a clinically viable system that adapts to changes in patients‘ EMG signals. At the end of this project we expect to have developed an adaptive neural control system that is ready for home-trials by injured service members.
MR140057
Development of an Engaging Training Tool to Provide Superior Muscle Computer Interfaces for Rehabilitation of Neuromusculoskeletal Injuries
The objective of this proposal is to develop an optimized pre-prosthetic training program that will increase the functional abilities of amputees and improve myoelectric prosthesis utilization.
Related Publications
Body
Spanias JA, Perreault EJ, and Hargrove LJ. A strategy for labeling data for the neural adaptation of a powered lower limb prosthesis. Engineering in Medicine and Biology Society (EMBC), 2014 36th Annual Intl Conf of the IEEE, 2014.
Ingraham KA, Fey NP, Simon AM, and Hargrove LJ. Contributions of knee swing initiation and ankle plantar flexion to the walking mechanics of amputees using a powered prosthesis. Eng in Med and Bio Society (EMBC), 2014 36th Annual Intl Conf of the IEEE, 2014.
Lenzi T, Hargrove LJ, and Sensinger JW. Preliminary evaluation of a new control approach to achieve speed adaptation in robotic transfemoral prostheses. Intelligent Robots and Systems (IROS 2014), 2014 IEEE/RSJ International Conference, 2014.
Lenzi T, Hargrove LJ, and Sensinger JW. Minimum jerk swing control allows variable cadence in powered transfemoral prostheses. Engineering in Medicine and Biology Society (EMBC), 2014 36th Annual Intl Conf of the IEEE, 2014.
Johnson RE, Kording KP, Hargrove LJ, and Sensinger JW. The effect of powered prosthesis control signals on trial-by-trial adaptation to visual perturbations. Engineering in Medicine and Biology Society (EMBC), 2014 36th Annual International Conference of the IEEE, 2014.
Gregg RD, Lenzi T, Hargrove LJ, and Sensinger JW. Virtual Constraint Control of a Powered Prosthetic Leg: From Simulation to Experiments With Transfemoral Amputees. IEEE Trans. Robot, 2014.
Young AJ, Kuiken TA, and Hargrove LJ. Analysis of Using EMG and Mechanical Sensors to Enhance Intent Recognition in Powered Lower Limb Prostheses. J Neural Eng, 2014.
Johnson RE, Kording KP, Hargrove LJ, and Sensinger JW. Does EMG Control Lead to Distinct Motor Adaptation?. Front Neurosci, 2014.
Fey NP, Simon AM, Young AJ, and Hargrove LJ. Controlling Knee Swing Initiation and Ankle Plantarflexion With an Active Prosthesis on Level and Inclined Surfaces at Variable Walking Speeds. IEEE J Transl Eng Health Med, 2014.
Birdwell JA, Hargrove LJ, Weir RF, and Kuiken TA. Extrinsic Finger and Thumb Muscles Command a Virtual Hand to Allow Individual Finger and Grasp Control. IEEE Trans Biomed Eng, 2014.
Albert MV, Deeny S, McCarthy C, Valentin J, and Jayaraman A. Monitoring Daily Function in Individuals with Transfemoral Amputations using a Commercial Activity Monitor: A Feasibility Study. PM&R, 2014.
Simon AM, Ingraham KA, Fey NP, Finucane SB, Lipschutz RD, Young AJ, and Hargrove LJ. Configuring a Powered Knee and Ankle Prosthesis for Transfemoral Amputees within Five Specific Ambulation Modes. Plos One, 9(6):e99387, 2014.
Wurth S and Hargrove LJ. A Real-time Comparison between Direct Control, Sequential Pattern Recognition Control and Simultaneous Pattern Recognition Control using a Fitts' Law Style Assessment Procedure. J NeuroEng Rehabil, 11(1):91, 2014
Hargrove L J, Simon AM, Young AJ, Lipschutz RD, Finucane SB, Smith DG and Kuiken TA. Robotic Leg Control with EMG Decoding in an Amputee with Nerve Transfers. New England Journal of Medicine, 369(13), 1237-1242, 2013.
Hargrove LJ, Simon AM, Lipschutz RD, Finucane SB, and Kuiken TA. Real-time myoelectric control of knee and ankle motions for transfemoral amputees. J AMA, 304(15):1542-1544, Apr 2011.
Huang H, Zhang F, Hargrove L, Dou Z, Rogers D, and Englehart K. Continuous locomotion mode identification based on neuromuscular-mechanical fusion for prosthetic legs. IEEE Trans Biomed Eng, 58(10):2867–2875, 2011